2021 AI-Powered Drug Discovery Symposium
-
You must log in to register
- Non-member - $50
- Member - $25
- Student Member - $25
- Lab Member - $25
Learn more about the various AI tools available today as well as discuss future drug discovery collaborations involving the cutting-edge advances in AI, machine learning and computational science.
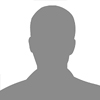
Daniel Ting, M.B.B.S., Ph.D.
Director of Singapore Health Service (SingHealth) AI Program, Head of AI and Digital Innovation
Singapore National Eye Centre
Associate Professor Daniel Ting is the Director of Singapore Health Service (SingHealth) AI Program, Head of AI and Digital Innovation in Singapore Eye Research Institute (SERI), Associate Professor in Ophthalmology with Duke-NUS Medical School Singapore. At the global setting, Dr Ting serves in several AI executive committee (American Academy of Ophthalmology, STARD-AI, QUADAS-AI, DECIDE-AI), AI editorial boards (Associate Editors for Nature Digital Medicine, Frontiers in Medicine and Frontiers in Digital Health; and Chair of AI and Digital Innovation Standing Committee, Asia-Pacific Academy of Ophthalmology. In 2017, he was also the US-ASEAN Fulbright Scholar who visited the Johns Hopkins University to deepen the AI collaboration between US and the ASEAN regions.
To date, he has published >200 peer-reviewed papers in highly prestigious journals such as JAMA, NEJM, Lancet, Nature Medicine, Nature Biomedical Engineering, Lancet Digital Health and etc. In 2021, he is also ranked the world’s most influential deep learning researcher across all domains in healthcare for the past 10 years (2010-2021) by the ExpertScape.
For the accomplishment, Dr Ting was recognized by many top-tiered international AI and ophthalmology societies in winning many prestigious scientific awards, including the MICCAI OMIA Prestigious Achievement Award (2020), ARVO Bert Glaser Award for Innovative Research in Retina (2020), USA Macula Society Evangelos Gragoudas Award (2019), APAO Young Ophthalmologist’s Award (2018), APTOS Young Innovator Award (2017) and etc.
With the advancement of AI technology, Daniel hopes to harness the power of digital technology, including big data, deep learning and blockchain, to improve patients’ outcome, experience, reduce health economic burden and more importantly, narrow the gap of inequality of health care access, standard and delivery globally.
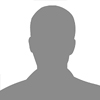
Kerry Gilmore
Assistant Professor of Chemistry
University of Connecticut
Dr. Kerry Gilmore is an Assistant Professor of Chemistry at the University of Connecticut. He grew up in Brewster, Massachusetts and attended Roger Williams University obtaining bachelors degrees in Biology and Chemistry. He received his Ph.D. in 2012 from Florida State University studying cyclization reactions, during which time he was a Fulbright Scholar working at the CNR in Bologna, Italy. He then moved to the Max-Planck Institute of Colloids and Interfaces for postdoctoral work, and was group leader of the flow chemistry group from 2014-2020. In 2020 he moved to the University of Connecticut where he is an Assistant Professor. His current research interests include the development of technology and approaches to advance methodologies of small molecule synthesis, as well as to utilize computational methods and machine learning to better understand and predict the selectivity of organic reactions. He recently won the ACS 2021 Award for Affordable Green Chemistry.
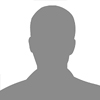
Ola Engkvist, Ph.D.
Head Molecular AI
AstraZeneca R&D
Dr Ola Engkvist is head of Molecular AI in Discovery Sciences, AstraZeneca R&D. He did his PhD in computational chemistry at Lund University followed by a postdoc at Cambridge University. After working for two biotech companies he joined AstraZeneca in 2004. He currently lead the Molecular AI department, where the focus is to develop novel methods for ML/AI in drug design , productionalize the methods and apply the methods to AstraZeneca’s small molecules drug discovery portfolio. His main research interests are deep learning based molecular de novo design, synthetic route prediction and large scale molecular property predictions. He has published over 100 peer-reviewed scientific publications. He is adjunct professor in machine learning and AI for drug design at Chalmers University of Technology and a trustee of Cambridge Crystallographic Data Center.
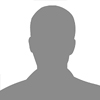
Rafael Gomez-Bombarelli, Ph.D.
Toyota Assistant Professor in Materials Processing
MIT DMSE
Rafael Gomez-Bombarelli is the Toyota Assistant Professor in Materials Processing in MIT’s Department of Materials Science and Engineering. Gomez-Bombarelli is interested in fusing machine learning and atomistic simulations for designing materials and their transformations. His group works across molecular, crystalline and polymer matter, combining novel computational tools in optimization, inverse design, surrogate modeling and active learning with simulation approaches like quantum chemistry and molecular dynamics. Through collaborations at MIT and beyond, his group helps develop new practical materials such as therapeutic peptides, organic electronics for displays, electrolytes for batteries, and oxides for sustainably catalysis. Gomez-Bombarelli’s work has been featured in the MIT Technology Review and Wall Street Journal. He also co-founded Calculario, a materials discovery company that leverages quantum chemistry and machine learning to target advanced materials in high-value markets. He earned a BS, MS, and PhD in chemistry from Universidad de Salamanca, followed by postdoctoral work at Heriot-Watt University and Harvard University.
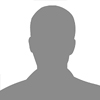
Payel Das, Ph.D.
IBM Research
Dr. Payel Das is a Principal Research Staff Member and a manager at IBM Research AI, IBM Thomas J Watson Research Center. She has also been an adjunct associate professor at the department of Applied Physics and Applied Mathematics (APAM), Columbia University. She received her Ph.D. on theoretical biophysics from Rice University, Texas. Currently, she leads research on trustworthy AI in the low-data regine and machine creativity. A central focus is on developing controllable generative AI and efficient black-box optimization techniques. The goal is to enable reliable modeling of complex systems and efficient synthesis of novel and useful designs for various downstream business and scientific applications, including drug discovery and material design.
Das has co-authored over 40 peer-reviewed publications and several patent disclosures, given dozens of invited talks at several university colloquiums, department seminars, top rated conferences, and workshops. She served as the editorial advisory board member of the ACS Central Science journal. She is the recipient of two IBM Outstanding Technical Achievement Awards (the highest technical award at IBM), two IBM Research Division Awards, one IBM Eminence and Excellence Award, and six IBM Invention Plateau Awards.
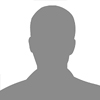
Dean Ho, Ph.D.
Head, Department of Biomedical Engineering
National University of Singapore
Provost’s Chair Professor
Director, The N.1 Institute for Health (N.1)
Director, The Institute for Digital Medicine (WisDM)
Head, Department of Biomedical Engineering
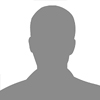
iao Liu, M.B.ChB., Ph.D.
Ophthalmologist
University Hospitals Birmingham NHS Foundation Trust
Dr Xiao Liu is an ophthalmologist and a post doctoral researcher at the University of Birmingham and University Hospitals Birmingham NHS Foundation Trust. She is interested in evidence standards for AI in healthcare, to ensure AI innovations can safely and effectively improve patient care. Xiao co-led the development of SPIRIT-AI and CONSORT-AI, the first international reporting standards for clinical trials of AI interventions, and is working with other AI reporting standards in development, including STARD-AI, DECIDE-AI and TRIPOD-AI. She also works with regulatory and commissioning bodies, including MHRA, NICE, the UK National Screening Committee and the WHO/ITU AI4H focus groups, on their approaches to evaluating AI in healthcare.
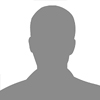
Edward Chow, Ph.D.
Editor-in-Chief, SLAS Technology; Associate Professor Cancer Science Institute of Singapore
National University of Singapore
Associate Professor Edward Kai-Hua Chow is a Principal Investigator at National University of Singapore (NUS) in the Cancer Science Institute of Singapore and the Department of Pharmacology. He is also the Research Director for the Institute of Digital Medicine (WisDM) and Dept. of Pharmacology. He received his B.A. in Molecular and Cellular Biology from the UC Berkeley and his Ph.D. at UCLA. Prior to joining NUS, A/P Chow was an American Cancer Society Postdoctoral Fellow under the guidance of Prof. J. Michael Bishop (1989 Nobel Prize in Physiology or Medicine) at UCSF. His research group is interested in understanding how to treat specific oncology patients or patient subtypes as well as the oncogenic drivers that determine subtype-specific therapy. In particular, his group is interested in applying engineering-based analytics towards personalised and precision drug combination design. Through the development of quadratic phenotypic optimisation platform (QPOP), his group has demonstrated that drug combination design for specific disease indications as well as specific patient groups can be quickly achieved in a cost, time and sample efficient manner. With an emphasis on haematological malignancies and gastrointestinal cancers, this work has been translated into multiple clinical studies as well as part of the NUS start-up, KYAN Therapeutics.
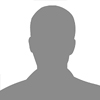
Elodie Pronier, Ph.D.
Lead Biologist
Owkin
Elodie Pronier, PhD, Lead Biologist, Owkin graduated from Université Denis Diderot (Paris 7) with a European Magister in Genetics, before completing her PhD in Oncology and Hematology at Institut Gustave Roussy. There, she focused on epigenetic mutations involved in leukemia development. Elodie then moved to New York as a postdoctoral research fellow at the Memorial Sloan Kettering Cancer Research in Ross Levine’s laboratory, where she co-led several projects on the genetics of leukemia. She is now a Lead Biologist within Owkin’s Biotech team. She is in charged of creating our early drug discovery pipeline.
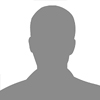
Lukas Pelkmans
Endowed Research Chair
University of Zurich
Lucas Pelkmans studied Medical Biology at the University of Utrecht in The Netherlands, and did his PhD in Biochemistry at the ETH Zurich in Switzerland. He was then a postdoctoral fellow at the Max Planck Institute of Molecular Cell Biology and Genetics in Dresden, Germany. In 2005, Lucas Pelkmans returned to Switzerland to start his research group in image-based systems biology at the ETH Zurich as an assistant professor, and was elected to the Ernst Hadorn Foundation-endowed Chair at the University of Zurich in 2010, where he has his research group since then. Lucas Pelkmans has received multiple awards for his pioneering work to establish the field of image-based systems biology, has received junior, consolidator, and advanced ERC grants, and is a member of EMBO.
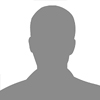
Leonidas Bleris, Ph.D.
Cecil H. and Ida Green Chair in Systems Biology Science and Professor
The University of Texas at Dallas
Leonidas Bleris is a Cecil H. and Ida Green Chair in Systems Biology Science and Professor with the Bioengineering Department of the University of Texas at Dallas. Before joining UTD, Bleris was a Postdoctoral Fellow at the FAS Center for Systems Biology at Harvard University. Bleris earned a Ph.D. in Electrical Engineering from Lehigh University in 2006. He received a Diploma in Electrical and Computer Engineering in 2000 from Aristotle University of Thessaloniki, Greece. Bleris was awarded the Christine Mirzayan Science and Technology Policy Graduate Fellowship from the National Academy of Science (NAS), and served with the Board of Mathematical Sciences and their Applications. During 2009-2010, Bleris was a Visiting Scientist at the FAS Center for Systems Biology at Harvard University, and an Independent Expert with the European Commission under the "Science, Economy and Society" directorate. Bleris served as the University of Texas at Dallas Representative for the 2011-2012 Tuning Oversight Council for Engineering and Science, Committee on Bioengineering, and received the 2014 Junior Faculty Research Award from the Erik Jonsson School of Engineering and Computer Science. In 2018 Bleris was awarded a Cecil H. and Ida Green Chair in Systems Biology Science. His research has focused on systems biology, mammalian synthetic biology and genome editing.
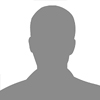
Chris Bakal
Professor of Cancer Morphodynamics
Institute of Cancer Research
Chris Bakal is the Professor of Cancer Morphodynamics at the Institute of Cancer Research in London, UK, where he leads the Dynamical Cell Systems Laboratory. His team aims to understand how cancer is driven by changes in cell shape.
Chris was born in Calgary, Canada. He received his BSc in Biochemistry from the University of British Columbia, and his PhD in Medical Biophysics from the University of Toronto. Chris’ postdoctoral work was performed in Department of Genetics at Harvard Medical School, and the Computer Science and Artificial Intelligence Laboratory (CSAIL) at the Massachusetts Institute of Technology (MIT). In 2007, Chris was named as one of the most promising postdoctoral fellows or junior faculty members at Harvard Medical School by the Dorsett L. Spurgeon award. After being awarded a Wellcome Trust Career Development Fellowship, Chris established his laboratory at the Institute of Cancer Research in London in 2009. In 2015 he was awarded the prestigious Cancer Research UK Future Leaders Prize.
Outside of science Chris is competitive track cyclist, a former national-level runner, and a former world-ranked downhill ski racer. Chris has run a mile in just over 4 min, and aims to compete in the Ironman next year.
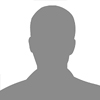
Finton Sirockin, Ph.D.
Associate Director
Novartis
Finton Sirockin, PhD, is Associate Director in Global Discovery Chemistry in Novartis, based in Basel.
Finton is a theoretical chemist / molecular modeller by training with a keen interest in exploring how data science can make drug discovery more efficient.
He obtained his Ph D. in Theoretical Chemistry from the University Louis Pasteur of Strasbourg in Prof. Martin Karplus group under the supervision of Prof. Annick Dejaegere, in 2003. After a year of Postdoc working on structural aspects of bioinformatics he joined Novartis’ CADD group in Basel. He delved into cheminformatics and machine learning through their application in MedChem projects.
He is providing MedChem project support in different Disease Areas. Since 2020, he is leading the Project Digital Lead initiative which fosters Data Science and Digital Tools literacy in the Medicinal Chemistry community in NIBR. He is also providing CADD support in MedChem projects and a machine learning collaboration applied to chemistry. Finally he is co-leading, at the MedChem project application level, the Generative Chemistry exploration collaboration between Microsoft Research and Novartis.
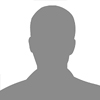
David Leslie
Ethics Lead
The Alan Turin Institute
Dr David Leslie is the Ethics Theme Lead within the public policy programme at the Alan Turing Institute. He is the author of the UK Government’s official guidance on the responsible design and implementation of AI systems in the public sector, Understanding artificial intelligence ethics and safety (2019) and a principal co-author of Explaining decisions made with AI (2020), a co-badged guidance on AI explainability published by the Information Commissioner’s Office and the Alan Turing Institute. He is PI of a UKRI-funded project called PATH-AI: Mapping an Intercultural Path to Privacy, Agency and Trust in Human-AI Ecosystems, which is a research collaboration with RIKEN, Japan, as well as of a BEIS and GPAI funded research project entitled Advancing Data Justice Research and Practice. David is also lead author of “Does AI stand for augmenting inequality in the COVID-19 era of healthcare” (2021) published in the British Medical Journal and the lead author of Artificial intelligence, human rights, democracy, and the rule of law (2021), a primer translated into Dutch and French. His other recent publications include the Harvard Data Science Review article, “Tackling COVID-19 through responsible AI innovation: Five steps in the right direction,” (2020), Understanding bias in facial recognition technologies (2020), and “The Arc of the Data Scientific Universe,” (2021) also published in the HDSR.
Before joining the Turing, David taught at Princeton’s University Center for Human Values. Prior to teaching at Princeton, David held academic appointments at Yale and at Harvard, where he received over a dozen teaching awards. He now serves as an elected member of the 9-person Bureau of the Council of Europe’s Ad Hoc Committee on Artificial Intelligence (CAHAI). He is on the editorial board of the Harvard Data Science Review and is a founding editor of the Springer journal, AI and Ethics.
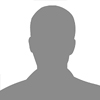
Brent Mittelstadt, Ph.D.
Senior Research Fellow
University of Oxford
Brent Mittelstadt is a Senior Research Fellow in data ethics at the Oxford Internet Institute. He is an ethicist and philosopher focusing on auditing, interpretability, and ethical governance of complex algorithmic systems, as well as data protection, privacy, and non-discrimination law. He coordinates the Governance of Emerging Technologies (GET) research programme at the OII, which investigates ethical, legal, and technical aspects of AI, machine learning, and other emerging technologies.
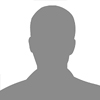
Carlos Maria Galmarini, M.D., Ph.D.
CEO
Topazium Artificial Intelligence
Carlos María Galmarini is Topazium’s founder. He earned his Medical Degree from the University of Buenos Aires (Argentina), after which he completed an internship in clinical oncology at Marie Curie Cancer Center (Buenos Aires). He pursued two post-doctoral fellowships in oncology at Los Angeles City of Hope Cancer Center (USA) and at Léon Bérard Cancer Center (Lyon, France). He obtained his PhD in oncology and his qualification to lead research teams (HDR) at Claude Bernard Lyon-1 University (Lyon, France). Carlos María practiced medicine both at the Marie Curie and Carlos G. Durand Hospitals in Buenos Aires for several years. He has also held positions as a medical researcher in oncology at Lyon Rockefeller Medical School and in Lyon-Sud Medical School (France), where he was appointed Associate Professor. In 2008 he joined PharmaMar (Madrid, Spain) as Head of Cell Biology and Pharmacogenomics, where he was in charge of drug screening, biochemistry, molecular biology and pharmacogenomics areas. He has authored over 130 publications across leading international scientific journals. In recent years, he devoted his research to the application of artificial intelligence in healthcare, molecular biology and other related-medical fields, particularly, in the generation of diagnostic assistance and predictive tools applied to clinical trials and real-world data.
Key:




