2019 AI in Process Automation Symposium
-
You must log in to register
- Non-member - $50
- Member - $25
- Student Member - $25
- Lab Member - $25
The SLAS 2019 AI in Process Automation Symposium course package contains 18 presentations on:
AI in Drug Discovery Today
Data Automation
AI in Screening
AI in Chemistry
Based on presenter permission, 18 of the 23 total SLAS 2019 AI in Process Automation Symposium presentations are available on-demand. The SLAS AI in Process Automation Program Committee selects conference speakers based on the innovation, relevance and applicability of research as well as those that best address the interests and priorities of today’s life sciences discovery and technology community. All presentations are published with the permission of the presenters.
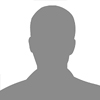
Alán Aspuru-Guzik, Ph.D.
Alán Aspuru-Guzik, Ph.D.
University of Toronto
Alán Aspuru-Guzik’s research lies at the interface of computer science with chemistry and physics. He works in the integration of robotics, machine learning and high-throughput quantum chemistry for the development of “self-driving laboratories”, which promise to accelerate the rate of scientific discovery. Alán also develops quantum computer algorithms for quantum machine learning and has pioneered quantum algorithms for the simulation of matter. Jointly appointed Professor of Chemistry and Computer Science at the University of Toronto. Previously, full professor at Harvard University. Co-founder of Zapata Computing and Kebotix, two early-stage ventures in quantum computing and self-driving laboratories respectively.
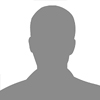
Jeremy Jenkins, Ph.D.
Executive Director
Novartis Institutes for BioMedical Research
Jeremy is Executive Director and Head of Data Science in Chemical Biology & Therapeutics at the Novartis Institutes for BioMedical Research. He joined Novartis 16 years ago, following a postdoc at Harvard Medical School and a PhD in Molecular Genetics from The Ohio State University. His global data science team collaborates in early discovery areas of Computational Biology, Cheminformatics, Imaging, and Machine Learning. Jeremy received the 2011 Corwin Hansch Award for contributions to the field of QSAR, and is a recipient of the Novartis Leading Scientist award.
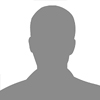
Casandra Mangroo, Ph.D.
Head of Science
BenchSci
Casandra is Head of Science at BenchSci. She applies research experience from her Ph.D. in Virology from the University of Toronto as the Science Team lead and product manager of BenchSci’s knowledge graph. Casandra is closely involved with developing the machine learning training sets and is responsible for the integrity and comprehensiveness of the scientific data on the BenchSci platform. She also works closely with BenchSci commercial leadership, R&D and engineering teams to implement new data initiatives, drive innovation and expand platform applications.
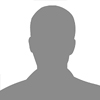
Casimir Wierzynski, Ph.D.
Senior Director AI Products Group
Intel
Casimir Wierzynski is Senior Director, Office of the CTO, in the Artificial Intelligence Product Group at Intel. He leads research efforts to identify, synthesize, and incubate emerging technologies that will enable the next generation of AI systems. Before joining Intel in 2017, Cas led research teams in neuromorphic computing and autonomous robotics at Qualcomm.
Cas received his BS and MS in electrical engineering at MIT, a BA in mathematics at Cambridge University as a British Marshall Scholar, and a PhD at Caltech in Computation and Neural Systems.
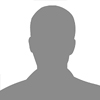
Steven Kuntz, Ph.D.
Sr. Scientist
Ionis Pharmaceuticals
Steven Kuntz conducts sequencing library creation, analysis, and protocol development at Ionis Pharmaceuticals in support of Functional Genomics and Drug Discovery. He received his PhD working on the robustness of muscle differentiation networks in the labs of Barbara Wold and Paul Sternberg at Caltech and did a postdoc on temperature-responsive developmental regulatory control with Michael Eisen at UC Berkeley.
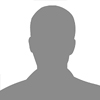
Joshua Kangas, Ph.D.
Assistant Teaching Professor
Carnegie Mellon University
A successful Computational Biology researcher will have a strong understanding of computational techniques and the biological processes and techniques underlying the development of the data they are analyzing. In the courses Joshua teaches at Carnegie Mellon University, students generate experimental data in wet-lab experiments and learn to apply computational techniques for the analysis of those data. Joshua constantly looks for ways to make our course offerings as modern as possible in both the wet-lab and computational techniques learned by students. At times, this has included modernizing classic labs or generating new modules based on novel research. He teaches courses for high school students, undergraduates, and graduate students (M.S. and Ph.D.).
He was also integral in the design and setup of the Automation Lab used by the M.S. Automated Science program at Carnegie Mellon University.
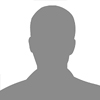
Juan Caicedo
Research Fellow
Broad Institute of Harvard
Juan Caicedo is a Research Fellow at the Broad Institute of MIT and Harvard, where he investigates the use of deep learning to analyze microscopy images. Previous to this, he studied object detection problems in large scale image collections also using deep learning, at the University of Illinois in Urbana-Champaign. Juan obtained a PhD from the National University of Colombia and completed research internships in Google Research, Microsoft Research, and Queen Mary University of London as a grad student, working in problems related to large scale image classification, image enhancement, and medical image analysis. His research interest include computer vision, machine learning and computational biology.
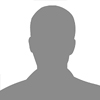
Oren Kraus, BASc, MASc, Ph.D.
Cofounder CTO
Phenomic AI
Oren Kraus co-founded Phenomic AI after completing his Ph.D. in Dr. Brendan Frey's lab at the University of Toronto. His research focused on applying deep learning to high-throughput microscopy screens used in drug discovery and cell biology research. Together with Jimmy Ba and collaborators at the Donnelly Centre for Cellular and Biomolecular Research (CCBR), Oren was one of the first to publish the application of deep learning to microscopy data. Oren founded Phenomic AI with the goal of accelerating the interpretation of phenotypes in bio-medical images with machine learning for applications in drug discovery and cellular diagnostics.
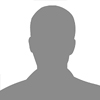
Lakshmi Akella, Ph.D.
Senior Scientist
Biogen
Lakshmi Akella is a computational chemist and is currently working as a Senior Scientist at Biogen in the Biotherapeutic & Medicinal Sciences Division. Prior to working at Biogen she worked at H3 Biomedicine, Broad Institute and Tripos.
She holds advanced degrees in Organic Chemistry & Software Engineering and conducted postdoctoral research at University of Minnesota.
She has several years of experience in enabling molecule design. She is specifically involved in ligand and structure-based design, virtual screening, library design, algorithm development, predictive modeling, high throughput screening technologies and cheminformatics in the industry.
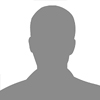
Stuart Chambers
Sr. Scientist
Amgen
Stuart Chambers is a Senior Scientist in the Genome Analysis Unit at Amgen with a background in stem cells, development, and neuroscience. His group uses induced pluripotent stem cells to model aspects of disease for drug development and works to create, evaluate, and disseminate technology for Amgen.
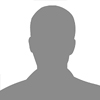
Connor Coley, Ph.D.
Postdoctoral Associate
MIT
Connor W. Coley is a postdoctoral associate at the Broad Institute of MIT and Harvard. His work in computer assistance and automation for organic synthesis has included the development of a data-driven synthesis planning program and in silico strategies for predicting the outcomes of organic reactions. For his work in this field, Connor has been named one of C&EN’s “Talented Twelve” and one of Forbes Magazine’s “30 Under 30” for Healthcare. His continuing research interests are in how data science and laboratory automation can be used to streamline discovery in the chemical sciences. He received his B.S. and Ph.D. in Chemical Engineering from Caltech and MIT, respectively. In 2020, he will return to MIT as an Assistant Professor in the Department of Chemical Engineering.
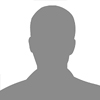
Sebastian Steiner, Ph.D.
Postdoctoral Research Fellow
University of British Columbia
I completed my MSc degree in Vienna, Austria, in the field of natural product synthesis, and then moved on to do my PhD in Glasgow, Scotland, in synthesis automation. I am interested in designing tools and writing software to enable lab automation, with a special focus on robotic reaction monitoring. In my spare time I train Historical European Martial Arts (sword fighting!), and I enjoy cooking and baking.
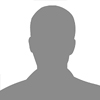
Jonathon Grob
Investigator
Novartis Institutes of Biomedical Research
I am a chemist who is passionate about leveraging my experience in Medicinal Chemistry & technology development to instigate a transformation toward automation & digital enabled drug hunting. 18 years at Novartis. Worked at 3 sites in my first 4 years. Currently working with a global group of entrepreneurial, automation loving, data science enabled team members to deliver MicroCycle 1.0 a new integrated drug discovery platform for NIBR.
Key:




